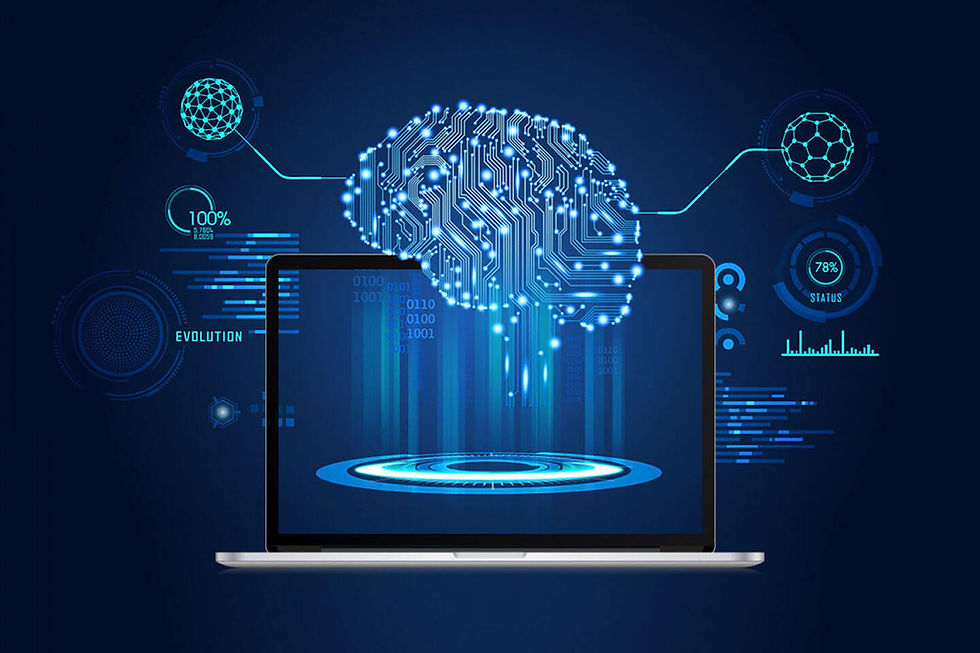
This transformative technology has revolutionized the field of language translation, reshaping the way we communicate across linguistic boundaries. By harnessing the power of deep learning, NMT has overcome numerous challenges that traditional rule-based and statistical methods struggled to conquer. In this blog, we delve into the journey of NMT and explore how deep learning has spearheaded a new era of accurate and nuanced language translation.
The Evolution of Machine Translation
Before the emergence of NMT, machine translation relied on rule-based systems and statistical models. Rule-based systems utilized predefined linguistic rules and dictionary-based translations, often resulting in translations that lacked fluency and failed to capture context. Statistical methods, on the other hand, utilized large parallel corpora to infer translation probabilities. While this approach improved fluency, it still faced difficulties with idiomatic expressions and context-sensitive translations.
The Rise of Neural Machine Translation
Enter Neural Machine Translation. The breakthrough of NMT can be attributed to the advent of deep learning, a subset of AI that involves complex neural networks inspired by the human brain's structure. Unlike previous approaches, NMT leverages neural networks to learn the relationships between words and phrases in different languages, capturing nuances and context in a remarkably human-like manner.
How NMT Works
NMT operates on the principle of sequence-to-sequence learning. It consists of two main components: an encoder and a decoder. The encoder processes the source language input and transforms it into a continuous vector representation called the "thought vector." This vector captures the semantic meaning of the input sentence. The decoder then uses this vector to generate the target language translation step by step, producing fluent and contextually accurate translations.
The Transformer Architecture
At the heart of NMT's success is the Transformer architecture. Introduced in a groundbreaking paper by Vaswani et al. in 2017, the Transformer model tackled the limitations of previous sequence-to-sequence models. It introduced the attention mechanism, allowing the model to focus on different parts of the input sequence as it generates the translation. This attention mechanism is the key to capturing long-range dependencies and understanding the context of the translation.

Advantages of NMT
Contextual Understanding
One of the standout features of NMT is its ability to understand context. By processing entire sentences at once, NMT models can grasp the nuances of idiomatic expressions and produce translations that retain the original meaning.
End-to-End Learning
Unlike traditional methods that required multiple stages of processing, NMT is an end-to-end learning process. This means that the entire translation task is handled within a single neural network architecture. This streamlined approach simplifies the translation process and allows the model to optimize its performance holistically.
Handling Rare and Ambiguous Phrases
Deep learning techniques enable NMT to handle rare and ambiguous phrases more effectively. Previous methods often struggled with out-of-vocabulary words or phrases that didn't have direct equivalents in the target language. NMT's continuous vector representations help bridge these gaps by mapping similar concepts onto each other.
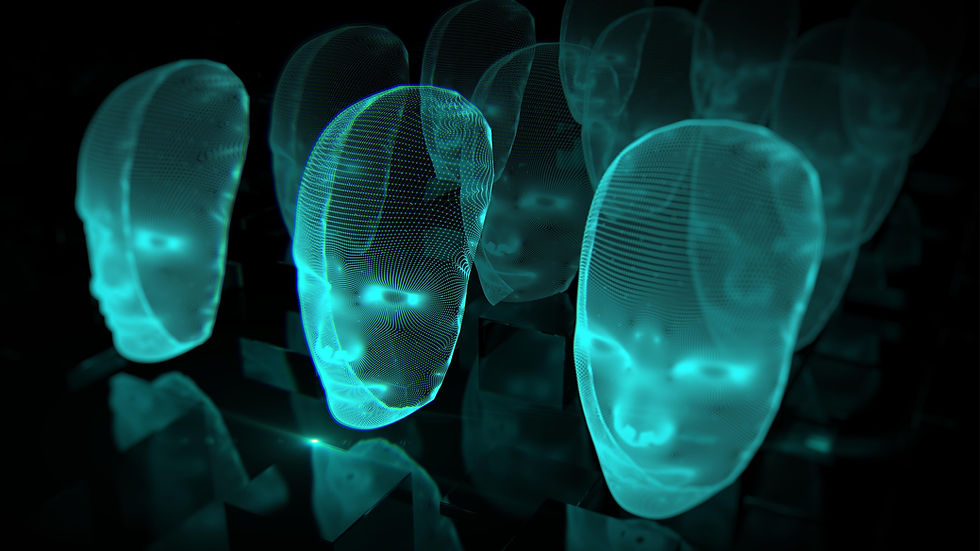
Challenges and Future Prospects
While NMT has transformed language translation, it is not without its challenges. The quality of translations can still be affected by biases present in the training data, and certain languages with limited parallel corpora may not benefit as much. Moreover, NMT models can sometimes produce overconfident or incorrect translations.
Looking ahead, researchers are focusing on improving low-resource language translation, reducing biases, and enhancing the robustness of NMT systems. Additionally, there is increasing interest in integrating NMT with other AI technologies, such as speech recognition and sentiment analysis, to create more context-aware and accurate translations.
In conclusion, Neural Machine Translation stands as a testament to the power of deep learning in reshaping technological landscapes. By learning from vast amounts of linguistic data, NMT models have transcended the limitations of previous approaches and are now providing us with more accurate, fluent, and nuanced translations. As researchers continue to refine and expand the capabilities of NMT, we can expect even more profound transformations in the way we communicate across languages, fostering global collaboration and understanding.
Comments